As the popularity of AI writing tools, such as ChatGPT, continues to grow, concerns around how to tackle the use of these tools in student submissions, too, continues to mount. Most educators around the globe are embarking on a new school year with both excitement and trepidation wondering how this school year will look in the age of AI?
Turnitin's AI writing detection solution aims to minimize false positives (incorrectly identifying fully human-written text as AI-generated), while still confidently identifying AI-generated content in submitted texts. We understand that false positive predictions may impact students' academic standing and reputation, creating additional complexity and effort due to investigation of AI writing predictions instances.
Our AI Writing detector’s false positive rate is less than 1% for documents with 20% or more AI writing (our tests showed that in cases where we detect less than 20% of AI writing in a document, there is a higher incidence of false positives). To further ensure that we maintain a less than 1% false positive rate, we tested the detector on 800,000 academic writing samples that were written before the release of ChatGPT, which we view as a safeguard that students are not incorrectly accused of AI writing misconduct. Since we cannot mitigate the risk of false positives completely given the nature of AI writing and analysis, it is important that educators use the AI score to start a meaningful and impactful dialogue with their students in such instances.
In addition to concerns around false positives, more recently, there have been a number of papers and articles claiming that AI writing detection tools are biased against writers for whom English is not their first language (also referred to as English Language Learner writers or ELL writers). Several of our customers have also reached out asking for deeper insights on how our model might address bias claims.
But before moving on to how we’ve tackled this issue of bias against ELL writers, we’d like to share some thoughts:
- The Turnitin AI detection system is trained on a representative sample of data that includes both AI-generated text and authentic academic writing across geographies and subject areas. While creating our sample dataset, we also took into account statistically under-represented groups like second-language learners, English users from non-English speaking countries, students at colleges and universities with diverse enrollments, and less common subject areas such as anthropology, geology, sociology, and others to minimize bias when training our model.
- Our evaluation of 800,000 documents—mentioned earlier in this blog—shows a slightly higher-than-comfortable false positive rate on short submissions (fewer than 300 words). There may not be enough signal/markers in such short samples to identify the telltale distributional differences in AI writing. In order to ensure we keep our false positive rate below 1%, we moved quickly to update the minimum submission length to 300 words for our AI writing detection capability to process a submission.
To that end, we want to come back to the claim that AI detectors demonstrate a common bias against ELL writers (Liang, 2023). Liang’s claim is grounded on a small collection of works of just 91 Test of English as a Foreign Language (TOEFL) practice essays, all of which are less than 150 words long. Turnitin's AI writing detection was not included in this evaluation, perhaps in part because we won't make predictions about documents that are so short.
Thus far, AI writing has been reasonably detectable because it's a little too probable, favoring repetition and unsurprising phrasing. "But wait!" you say, "isn't formulaic, repetitive writing a characteristic of developing writers, or English language learners? How can you tell AI writing apart from a basic, by-the-book essay?"
To answer this question, we decided to expand our false positive evaluation further to include ELL writers, using writing samples from a few open datasets (see details under the Dataset references section).
The objective of this test was to verify whether Turnitin’s AI writing detector shows a bias against ELL writers.
Process
Test dataset
We sampled up to 2,000 texts for each combination of "L2 English" or "L1 English" and "too short" (between 150 and 300 words) or "long enough" (300 words or more) from the below datasets, with permission from their rights-holders where applicable. These datasets are not included in our AI writing detector’s training set—we’re only using them for this public evaluation. Most of the L2 English documents are short persuasive or informative writing tasks, most similar to the Automated Scoring and Assessment Prize (ASAP) secondary-level essay corpus. A comparable collection of publicly available full-length university level documents from L2 and L1 writers was not available for this evaluation.
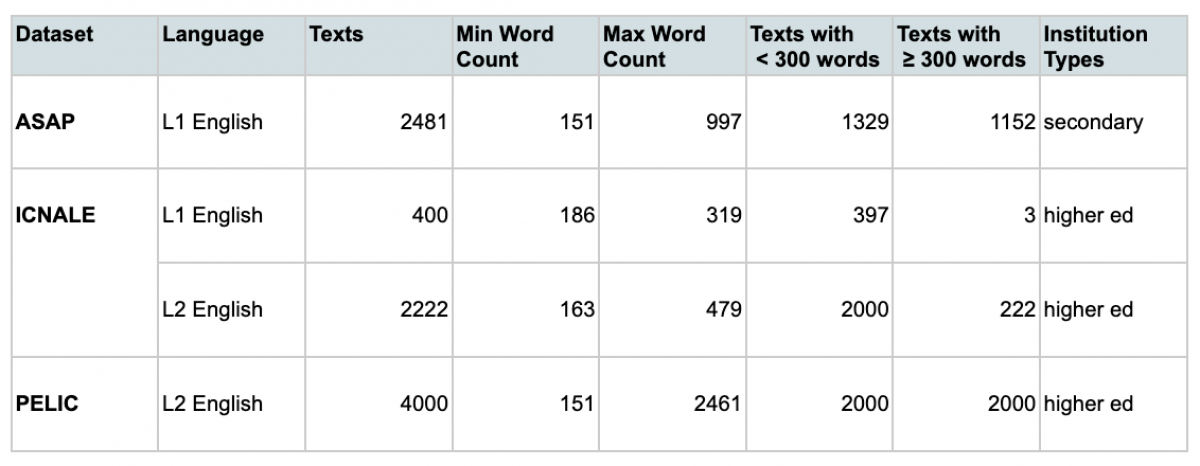
We evaluated each text in our combined dataset using the current version of Turnitin’s AI writing detector available to our customers, modified only to permit predictions on documents with fewer than 300 words (since our detector has a minimum word count threshold of 300 words).
Results
For documents meeting our minimum 300 word count requirement, the difference in the false positive rate (FPR) between L2 English writers (ELL writers) and L1 English writers (native English writers) is small, and not statistically significant, illustrating that our detector is not biased between these two groups of writers. Additionally, although the FPR for each group is slightly higher than our overall target of 0.01 (1%), neither group’s FPR is significantly different from this target.
Conversely, for documents that are “too short,” we found there is a greater difference in FPR between these groups of writers and it’s significantly greater than our target of 0.01 FPR. This again corroborates our earlier finding that AI writing detectors require longer samples to correctly identify AI-generated content and to keep false positives to a minimum.
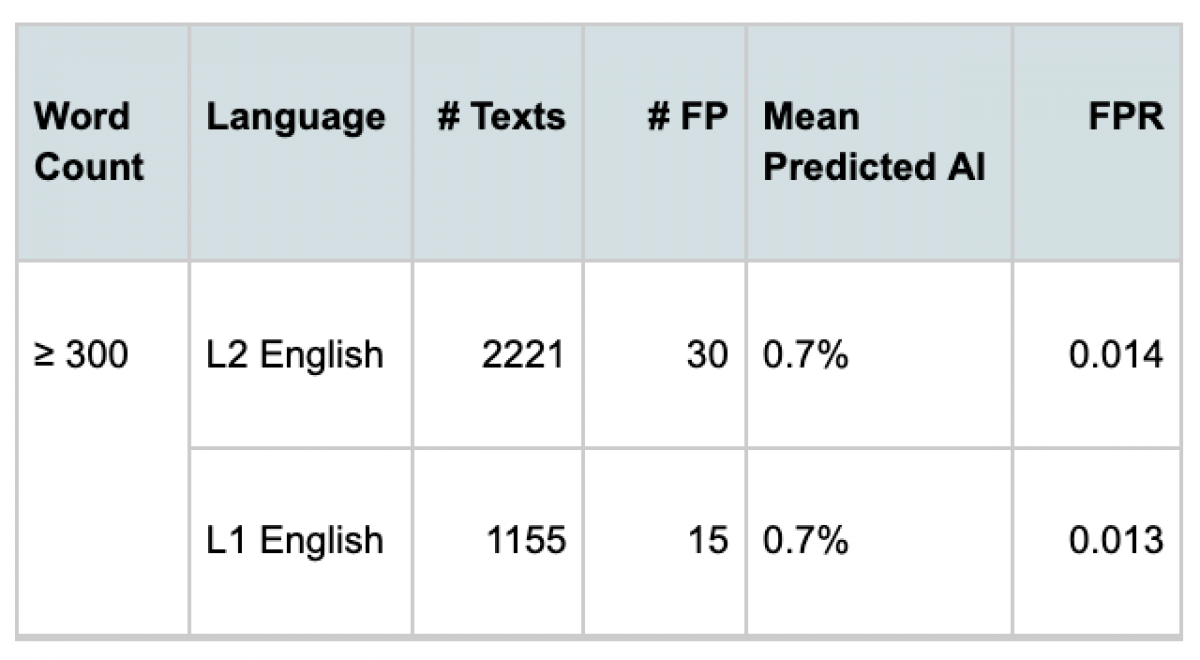
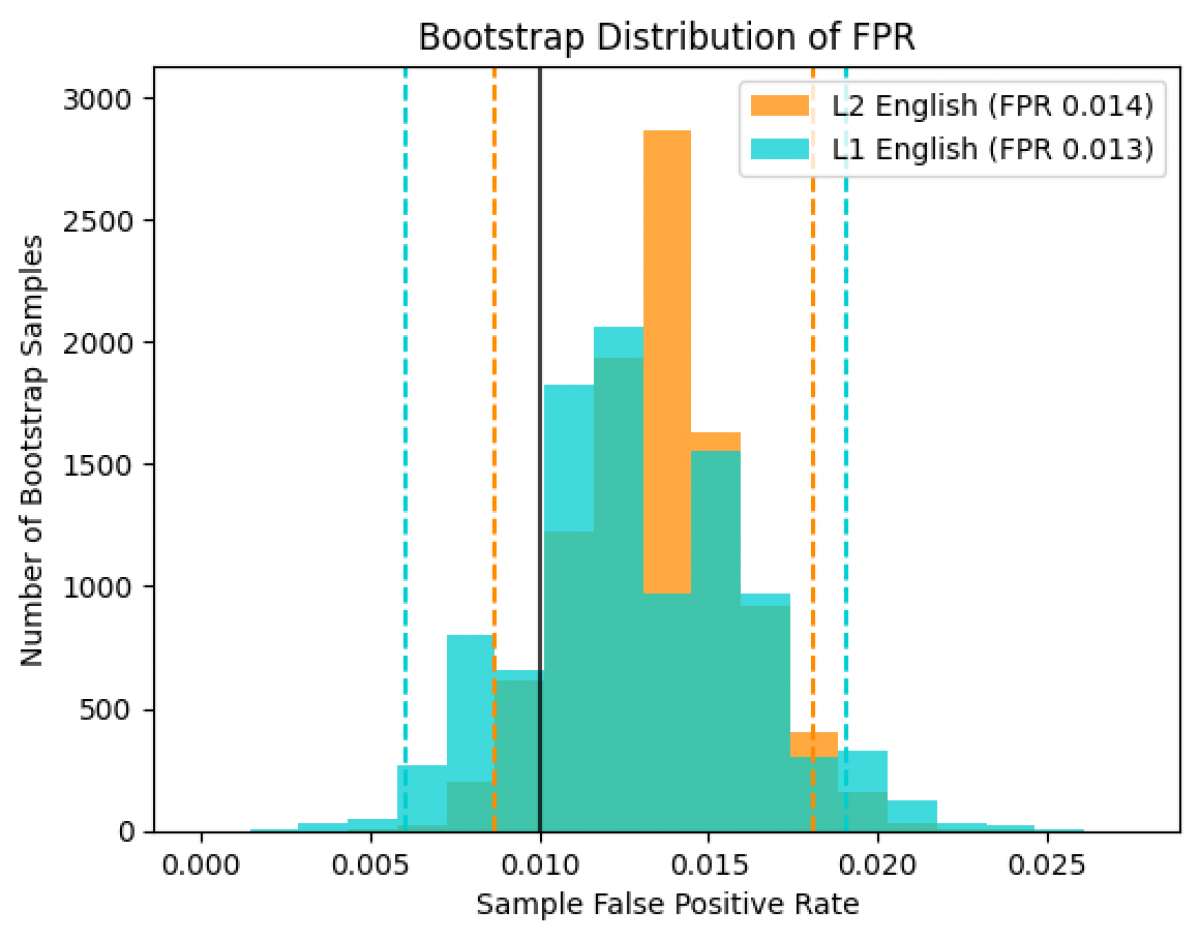
Bootstrap distribution of False Positive Rate among documents with at least 300 words. Dashed lines indicate the 95% confidence intervals for each group’s FPR. The solid line indicates our target FPR.
Conclusion
Based on this evaluation, the Turnitin AI writing detector does not appear to show a significant bias against writers for whom English is not their first language for submissions that meet our minimum word count requirement.
As LLMs continue to develop, we will continue to enhance and improve our AI writing detection capabilities to ensure we provide a safe and effective solution to our customers.
Works Cited
Liang, W., Yuksekgonul, M., Mao, Y., Wu, E., & Zou, J. (2023). GPT detectors are biased against non-native English writers. arXiv preprint arXiv:2304.02819.
Dataset references
- Juffs, A., Han, N-R., & Naismith, B. (2020). The University of Pittsburgh English Language Corpus (PELIC) [Data set]. http://doi.org/10.5281/zenodo.3991977
- This dataset was funded with a grant via the Pittsburgh Science of Learning Center, award number SBE-0836012. (Previously NSF award number SBE-0354420.)
- Ishikawa, S. (2023). The ICNALE Guide: An Introduction to a Learner Corpus Study on Asian Learners’ L2 English (Routledge).